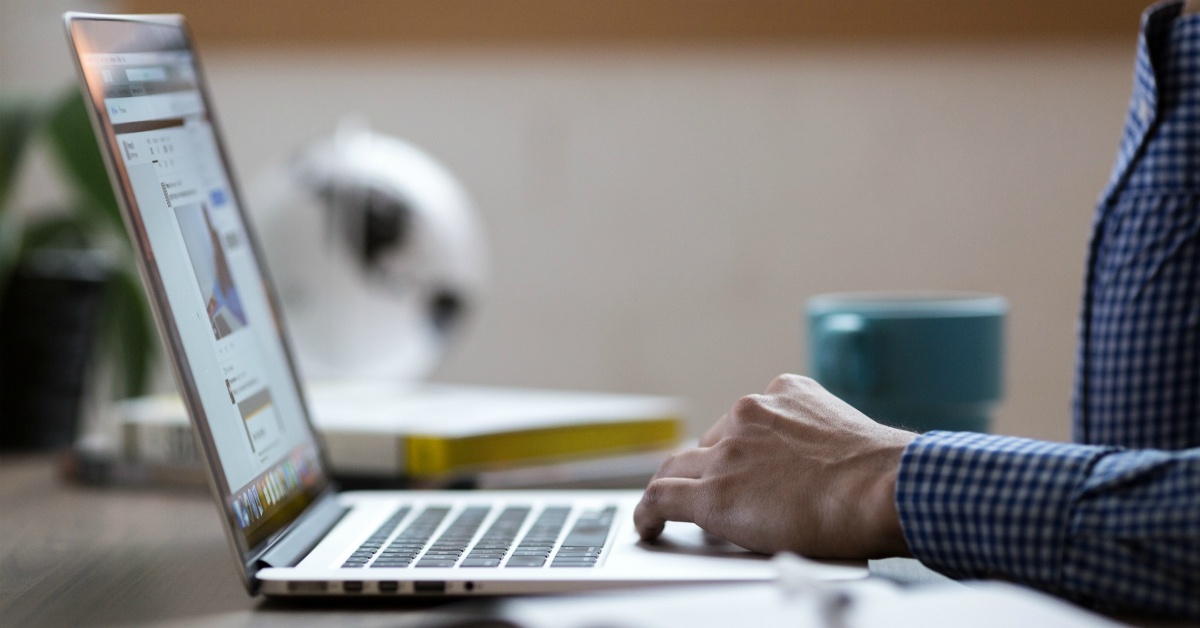
When I was in graduate school, I quickly gravitated to projects and classes that focused on the relatively new field of database design and database technology. I loved the deep analysis of data and exploring the question of how to leverage technology to support storage and access to data in order to find answers. It was challenging, it was new, and it was a field that I knew would have a huge impact. My thesis was about data organization and optimization, and I was lucky to be able to experiment with all sorts of database challenges and software as I ultimately built my own contribution to the science.
At the Optum Forum conference this year, once again I was brought back to the data. As a recent partner with Optum, Healthmonix provides MACRA (MIPS and ACO) reporting for Optum clients in addition to our existing client base. At the conference, I heard the cries of how fundamental the data really is as we move forward in the value-based care market. As much as we need to work with providers and payers to change patterns of practice, a critical component is the data that supports the change and that measures the impact.
This is because data is what drives precision medicine and AI initiatives. It drives understanding, affirms what we already know, points out new patterns that we haven’t realized, and shows us where our perceptions are correct and where they are not.
Data can be difficult.
And believe me, gathering and formatting that data is a challenge with a capital ‘C’. Both in our company’s work and in Optum’s, the data must be normalized, scrubbed, coded, and organized—not to mention the technical hurdles of acquiring it in the first place. Optum indicates that for clients there is a significant implementation cycle needed to pull data and organize it into information that can be used to answer fundamental questions of patient characteristics, episodes, care patterns, care coordination, quality metrics, and cost.
At Healthmonix, last year we aggregated and analyzed data from 134 different EHRs. In each case the data is coming from a different structure, potentially with different meanings and created by different people. With the variety of systems that are utilized, we first look at the basic structure and the stated meaning of each field to determine the potential use. Then we look at the values to see if they make sense. If the data field is called ‘immunization’, it might hold ‘yes/no’ values or Snomed (or other coding system) codes or CVX codes for the actual vaccine, or other information. Therefore, each value needs to be scrutinized in order to join into a data store that can provide meaning when combined with other data. And we know that each provider / user of systems might be entering their data slightly (or very) differently.
Interoperability alone isn’t enough.
The term “interoperability” is often thrown around, but what would that look like if it actually existed? As noted above, even if the data flowed easily, there is little standardization. Therefore interoperability would only be a first step toward a robust data solution. We still need to evaluate, validate, transform and load the values that we find in our disparate systems. And more than likely, in order to accomplish this, there needs to be discussion between those involved in the data sources and those performing the translation and integration.
Healthmonix is committed to being part of the solution for the data issues in the current healthcare system. We work to increase the validity and the value of the data. Once this is done, the data can drive not only MACRA solutions, but also a myriad of other applications. But I’ll save those for another day.